Big Data & the Future of Selling ‘Stuff’
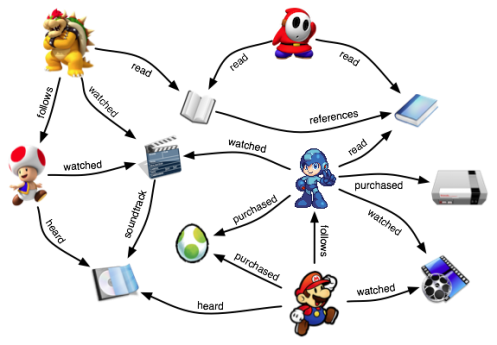
I don’t know if you read about this before the holidays, but I got to thinking about Amazon’s offer to pay shoppers $5 to use it’s mobile application to compare its prices to those in a store (this was a one-day promotion on Dec. 10 that provided 5% or up to $5 off as many as three items for customer’s efforts to price check).
Amazon is the leader in online retailing at a $81 Billion market cap (a little under 2x it’s annual revenue of $44 Billion)….and for a good reason. They understand their customers and their customer’s experiences better than anyone.
This promotion was an interesting use of consumer data entry to power next generation retail price competition. The promotion served as a way for Amazon to increase usage of its bar-code-scanning application, while also collecting intelligence on prices in the stores.
This is one of many interesting trends that is TERRIFYING brick-and-mortar retailers (large and small). While the real-time “Everyday Low Price” information empowers consumers to spend less, it terrifies retailers, who increasingly are feeling like showrooms — shoppers come to to check out the merchandise but ultimately decide to walk out and buy online instead.
It’s no surprise that Forrester predicted that this year, 2012, will mark the turning point for web-influenced retail sales (the online and mobile web will influence over half of overall retail sales). If we just look at US online purchases alone (excluding in-store), it’s a market exhibiting a 10% CAGR which will be $250 Billion by 2014. That’s not a bad place to be….assuming you have access to technologies similar to what Amazon uses when it comes to understanding customers.
So outside of moving your business online, what can these terrified brick-and-mortar retailers do?
I think it comes down to understanding their customer’s interests and providing them an outstanding experience. You don’t want to compete based on selection, volume, or price. If that’s your model, you’ve already lost the game.
And I’m not talking about the typical “online recommendation system” or “collaborative filtering” approach which can include algorithms of ‘Most Viewed,’ ‘Top Sellers,’ ‘People who bought or viewed this also bought that.’ I’m talking about actions that can be made both online AND offline that occur before, during, and after a desired purchase outcome.
Consumer Interest
Lets analyze Marko Rodriguez‘s “property graph” represented in the graphic above, or as others might describe, an Interest Graph. Om Malik at GigaOm spoke about this last year in reference to Hunch (who has recently been acquired by Ebay…go figure!). Prior to that, GigaOm reported on Interest Graph as it related to Gravity’s web offerings.
Today’s web recommendations are created as part of the process of mapping a user to a particular set of items. These items may be products (e.g. music, movies, games, books, accessories) or they may be people (e.g. find an expert, someone to follow).
Within any data set, there are numerous such user-to-item mappings. Typical recommendation mappings exploit co-interest patterns (e.g. collaborative filtering) or they exploit item feature patterns (e.g. content-based recommendation).
As Marko explains, there is more to recommendation than what is generally understood. For one, there are as many user-to-item mappings as there are paths through the data. “Paths?” When your data is modeled as a graph, the graph traversal pattern can yield a plethora of different mappings—and this collection can be used to soothe the needs of your users as their moods and whims change…and, ideally, in real-time (so it’s relevant).
As long as you have access to the data related to your customer’s entire experience, across as many interaction channels (Web, Advertising, Email, Social, Search, POS, etc.), an interest graph dataset (such as the one diagrammed above) can be created, and many types of recommendations can be made. Examples include, but are not limited to:
- Recommend people to follow based on similar behavior.
- Recommend people to follow based on similar movie watching behavior.
- Recommend products based on shared features.
- Recommend movies to watch based on reading interests.
- Recommend songs to listen to that are related to these particular followed people.
- Recommend books or movies that are similar to songs listened to today.
The question is whether you can seamlessly map your existing data sources (e.g. SQL databases, Apache web logs, etc), supplement with additional data (e.g. transcribed call-center logs), then discover the interest graph, and finally update/process it in real-time with “next best actions” which, ultimately, improves your customer’s experience.
Think of a new kind of commerce experience that goes beyond the notion of group shopping, shopping communities, and recommendation engines.
Think of a commerce experience, which not only takes into account your social graph, but also your interests graph.
Think of a commerce experience where we not only appreciate what our customers are looking for, but we also appreciate what happens after they get what they’re looking for….understanding your customer’s experiences before, during, and after their desired outcome…
After all, it’s all about the experience and not just buying ‘stuff’, right?
Great post Jim. Looking forward to meeting in person at Antonio’s at some point — though for some reason I thought you were in SoCal…
wow Jim; very cool thoughts and a powerful potential tool. where are you guys in terms of having a deployable tool? I’d be very interested in talking with you more about this. I’m wondering how such a tool could be deployed at Universal Sports (or any other large media company focused on driving increased impressions) to enhance consumer experience with a media website to improve customer stickiness and lengthen viewing or fan interaction times. I think I might be able to get you into some interesting conversations about deploying the tech this way if you have a product. Let’s discuss.